Data Science Meets AI: Understanding the Intersection
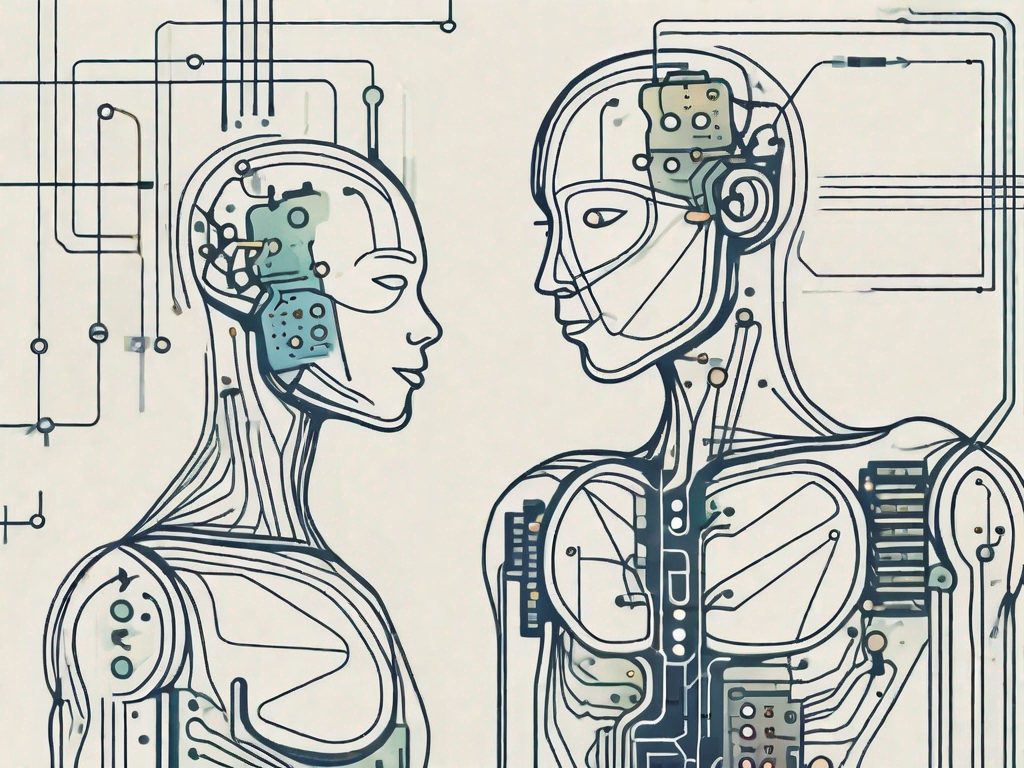
In the world of technology and innovation, two terms that have gained significant attention are Data Science and Artificial Intelligence (AI). These two fields have revolutionized industries and are driving the way we understand and utilize data. Understanding the intersection of Data Science and AI is crucial in today's data-driven world. This article aims to provide an in-depth understanding of the convergence of these two domains, their impact on each other, and the challenges and future trends associated with this synergy.
Defining Data Science and AI
To comprehend how Data Science and AI converge, let's first define these terms and understand their individual roles.
Data Science and Artificial Intelligence (AI) are two rapidly growing fields that have revolutionized the way we analyze and interpret data. These fields have become indispensable in various industries, including healthcare, finance, and technology.
The Core Principles of Data Science
Data Science is a multidisciplinary field that uses scientific methods, algorithms, and processes to extract insights from structured and unstructured data. It involves techniques such as data mining, statistical analysis, and machine learning to uncover meaningful patterns and make informed decisions.
Data Scientists are skilled professionals who possess a deep understanding of mathematics, statistics, and computer science. They have the ability to collect, clean, and transform raw data into a format that can be analyzed. By applying advanced analytical techniques, they can identify trends, predict future outcomes, and provide valuable insights to drive business growth.
One of the key aspects of Data Science is the utilization of machine learning algorithms. These algorithms enable computers to learn from data and make predictions or take actions without being explicitly programmed. By training models on large datasets, Data Scientists can create intelligent systems that can recognize patterns, classify information, and make accurate predictions.
The Fundamentals of Artificial Intelligence
Artificial Intelligence, on the other hand, refers to the simulation of human intelligence in machines that can perform tasks that would typically require human intelligence. AI encompasses various subfields such as natural language processing, computer vision, and robotics.
Natural Language Processing (NLP) focuses on enabling computers to understand and interpret human language. This field involves tasks such as speech recognition, sentiment analysis, and language translation. NLP algorithms enable machines to comprehend and respond to human language, making it possible for chatbots and virtual assistants to interact with users.
Computer Vision is another subfield of AI that deals with enabling machines to interpret and understand visual information. It involves tasks such as image recognition, object detection, and facial recognition. Computer Vision algorithms enable machines to analyze and interpret visual data, making it possible for self-driving cars to perceive their surroundings and for security systems to identify potential threats.
Robotics is a field that combines AI with engineering to create intelligent machines that can perform physical tasks. Robots equipped with AI capabilities can perform complex actions, interact with their environment, and adapt to changing circumstances. From manufacturing to healthcare, robots are being used to automate processes, assist in surgeries, and enhance overall efficiency.
By combining the principles of Data Science and AI, organizations can unlock the full potential of their data and leverage intelligent systems to gain a competitive edge. The convergence of these fields has paved the way for advancements in various domains, leading to improved decision-making, personalized experiences, and innovative solutions to complex problems.
The Convergence of Data Science and AI
Data Science and AI are interdependent and complement each other in multiple ways. Let's explore how these domains converge and share common ground.
When we delve into the convergence of Data Science and AI, we uncover a fascinating relationship that goes beyond mere complementarity. These two fields, although distinct, intertwine to create a powerful force that drives innovation and unlocks the potential of data-driven decision-making.
How Data Science Complements AI
Data Science provides the foundation for AI by enabling the collection, cleaning, and preparation of vast amounts of data. Without proper data handling and analysis, AI algorithms would lack the necessary input to make accurate predictions or decisions.
Consider a scenario where an AI system is designed to predict customer preferences for an e-commerce platform. In this case, Data Science plays a pivotal role in gathering and organizing customer data, such as purchase history, browsing behavior, and demographic information. Through data preprocessing techniques, Data Scientists ensure that the collected data is accurate, complete, and ready for analysis.
Moreover, Data Science techniques, such as data cleaning and feature engineering, enhance the quality of input data for AI algorithms. By removing outliers, handling missing values, and transforming variables, Data Scientists ensure that the data fed into AI models is reliable and representative of the real-world phenomena it aims to capture.
The Role of AI in Data Science
AI enhances Data Science by incorporating advanced algorithms and models that can process large volumes of data quickly. AI algorithms enable automated feature selection, exploratory data analysis, and predictive modeling, taking Data Science to new heights.
Imagine a Data Scientist tasked with analyzing a massive dataset containing millions of records. Without the aid of AI, this would be an arduous and time-consuming process. However, with the integration of AI techniques, such as machine learning and deep learning, Data Scientists can leverage algorithms that automatically identify relevant features, uncover hidden patterns, and generate accurate predictions.
Furthermore, AI empowers Data Scientists to explore complex relationships within the data and gain valuable insights. Through techniques like clustering, classification, and regression, AI algorithms enable Data Scientists to uncover meaningful patterns and make data-driven decisions with confidence.
In summary, the convergence of Data Science and AI creates a symbiotic relationship where each field strengthens and complements the other. Data Science provides the groundwork for AI, ensuring the availability of high-quality data, while AI enhances Data Science by enabling advanced analytics and predictive modeling. Together, they form a powerful duo that propels us into a future where data-driven innovation thrives.
The Impact of AI on Data Science Techniques
AI has revolutionized the way data is analyzed and interpreted. Let's delve into two critical areas where the impact of AI on Data Science is profound.
Machine Learning and Data Science
Machine Learning, a subset of AI, has become an integral part of Data Science. Machine Learning algorithms learn from data patterns and adapt autonomously, enabling Data Scientists to build accurate predictive models and discover valuable insights from complex datasets.
One example of the impact of Machine Learning on Data Science is in the field of healthcare. With the help of AI, Data Scientists are able to develop models that can predict the likelihood of a patient developing a certain disease based on their medical history, lifestyle choices, and genetic makeup. This has the potential to revolutionize preventive medicine, allowing doctors to intervene early and potentially save lives.
Another area where Machine Learning has made a significant impact is in the financial industry. AI-powered algorithms can analyze vast amounts of financial data in real-time, detecting patterns and anomalies that may indicate fraudulent activities. This has greatly enhanced the ability of financial institutions to detect and prevent fraud, protecting both the institutions and their customers.
Deep Learning in Data Analysis
Deep Learning, a subfield of Machine Learning, uses Artificial Neural Networks to analyze and make sense of vast amounts of unstructured data, such as images, audio, and text. This technique has revolutionized image and speech recognition, natural language processing, and sentiment analysis.
One fascinating application of Deep Learning in Data Analysis is in autonomous vehicles. AI-powered systems can analyze real-time data from sensors and cameras, allowing the vehicle to perceive its surroundings and make decisions accordingly. This has the potential to greatly improve road safety and reduce accidents caused by human error.
Another area where Deep Learning has shown remarkable results is in the field of drug discovery. By analyzing large datasets of molecular structures and their interactions with target proteins, AI algorithms can identify potential drug candidates with high efficacy and minimal side effects. This has the potential to accelerate the drug development process and bring life-saving medications to market faster.
In conclusion, AI has had a profound impact on Data Science techniques. Machine Learning has enabled Data Scientists to build accurate predictive models and discover valuable insights, while Deep Learning has revolutionized the analysis of unstructured data. As AI continues to advance, we can expect even more exciting developments in the field of Data Science.
Challenges and Solutions at the Intersection
Despite the numerous benefits of combining Data Science and AI, several challenges arise at this intersection.
Overcoming Data Quality Issues
Data Science and AI models heavily rely on high-quality data for accurate predictions and insights. Ensuring data quality by addressing issues like missing values, outliers, and data biases is crucial to maintain the integrity of the models.
Addressing Ethical Concerns in AI and Data Science
AI and Data Science come with ethical concerns such as privacy, bias, and transparency. It is essential to implement ethical guidelines, automate decision-making processes without violating individuals' rights, and ensure fairness and accountability in AI-driven applications.
Future Trends in AI-Driven Data Science
The synergy between Data Science and AI continues to evolve, giving rise to exciting future trends.
Predictive Analytics and AI
With the advancements in AI, predictive analytics is becoming increasingly accurate and efficient. AI-driven predictive models can anticipate future trends, behavior patterns, and customer preferences, enabling businesses to make proactive decisions and gain a competitive edge.
The Rise of Automated Machine Learning
Automated Machine Learning (AutoML) is revolutionizing the Data Science landscape. AutoML tools automate the selection of algorithms, feature engineering, and hyperparameter tuning, making Data Science more accessible to non-experts and speeding up the model development process.
Data Science and AI are no longer separate entities; they are entwined, complementing and elevating each other. Understanding the intersection of these domains is essential for professionals across various industries to make informed decisions, leverage the power of data, and embrace the opportunities that AI-driven Data Science offers.